Conference Program
The IWSSIP 2021 Conference program is available both online and as a downloadable PDF.
Invited Talks
We are happy to introduce our keynote speakers who will talk about the latest in their respective research areas.
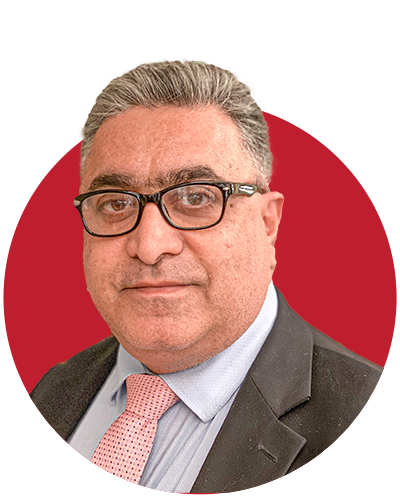
Touradj Ebrahimi
École Polytechnique Fédérale de Lausanne, Switzerland
Keynote Talk
JPEG AI:
Towards the First Learning based Image Compression Standard
The JPEG standardisation committee has been active in the last three decades as a major contributor to digital imaging ecosystem both in consumer and in professional markets. We start this talk with a quick overview of two JPEG standards that followed its first and most widespread image compression format, namely, JPEG 2000 and JPEG XR. Doing so, we discuss rationales for which JPEG 2000 and JPEG XR were defined and challenges they have faced since their release.
We then continue with a recent image coding initiative that aims at defining a new class of image compression suitable for both human and machine vision applications. After discussing the scope this effort, that is referred to as JPEG AI, its objectives and potential applications its aims for, we see the strategy that was defined and implemented by the JPEG committee in the past 3 years to define such a standard, what has been accomplished and what are the remaining challenges.
A particular emphasis will be put on how JPEG lied on contributions from academia, industry and users community, in order to define a solution that takes into account the views of all stakeholders. The talk will end by presenting how the same strategy used in JPEG AI is now being implemented in other JPEG standardisation activities such as JPEG Pleno Point Cloud Coding which will be also based on artificial intelligence.
Keynote Talk
Delivering High Quality Rich Media Content in Current Network Environment: Challenges and Solutions
More than three quarters of world network data traffic is already video-related. This content does not only consist of professional and user generated clips, video from streamed and downloaded services and media pre-recorded or generated on the fly, but also content with different degrees of interactivity. Rich media also includes multi-sensorial media, omnidirectional video and mixed reality content. This content can be consumed at home, at work, in public places by a heterogeneous user population, while static or on the move.
A wide range of devices contributes to generation and use of this content is being distributed over a network environment which is also highly heterogeneous. Challenges of transmitting this rich media content are highlighted and several solutions to support high quality, performance and/or energy efficiency of rich media delivery in such a complex context are presented.
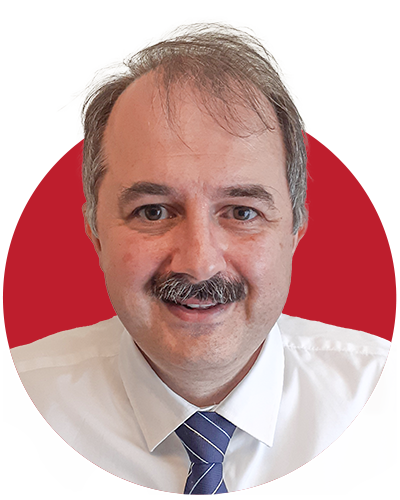
Gabriel Miro-Muntean
Dublin City University, Ireland
Prof. Gabriel-Miro Muntean is a Professor with the School of Electronic Engineering, Dublin City University (DCU), Ireland, where he obtained his PhD degree in 2004 for research on quality oriented adaptive multimedia streaming. Prof. Muntean is co-Director of the DCU Performance Engineering Laboratory and investigator of Science Foundation Ireland Insight and Lero National Research Centres. His research interests include quality-oriented and performance-related issues of adaptive rich media delivery, performance of wired and wireless communications, energy-aware networking and performance of large scale networking systems.
Prof. Muntean has published over 450 papers in prestigious international journals and conferences, has authored 4 books and 23 book chapters and has edited seven other books and conference proceedings, which attracted over 7500 citations (H-index 48). Prof. Muntean is Associate Editor of the IEEE Transactions on Broadcasting, Multimedia Communications Area Editor for the IEEE Communication Surveys and Tutorials and reviewer for other important international journals, conferences and funding agencies. He is a senior member of IEEE and IEEE Broadcast Technology Society. He was the coordinator of the EU Horizon 2020 project NEWTON (http://newtonproject.eu) and is DCU coordinator for the EU Horizon 2020 project TRACTION (https://www.traction-project.eu).
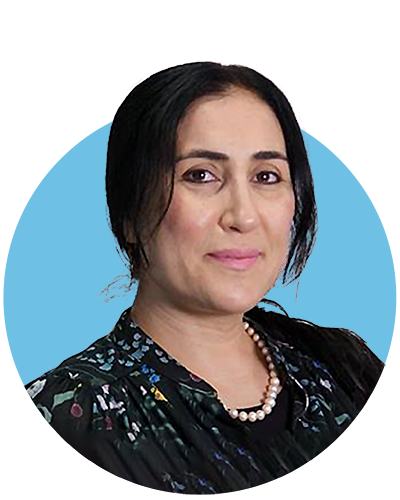
Abir Hussain
Liverpool John Moores University, United Kingdom
Abir Hussain is a professor of Machine Learning and a senior member at the Faculty of Engineering and Technology.
She completed her PhD study at The University of Manchester (UMIST), UK in 2000 with a thesis title Polynomial Neural Networks for Image and Signal Processing. She has published numerous referred research papers in conferences and Journal in the research areas of Neural Networks, Signal Prediction, Telecommunication Fraud Detection and Image Compression.
She worked with recurrent neural networks and deep learning techniques and their applications to image and signal processing. Abir has published in a number of high esteemed and high impact journals including IEEE Access, Pattern Recognition Letters, IEEE Internet of Things Magazine, Ad Hoc Networks, IEEE Transactions on Sustainable Computing, Expert Systems with Applications, PloS ONE, Electronic Letters, Neurocomputing, and Neural Networks and Applications.
Abir has successfully supervised 20 research students and has been an external examiner to various UK and overseas Universities for postgraduate research degrees.
She has been actively involved as a member of editorial board and review committee for a number peer reviewed international journals including Neural Networks and Plos One.
Keynote Talk
Detection and Localization of Objects within Images Using Computer Vision and Machine Learning
Data Science represents a multidisciplinary field that involves using various techniques including pattern recognition, computer programmed algorithms and image analysis. knowledge can be gained through datasets gathered from various resource and with various sizes. Mobile phones, remote sensors, software logs and social media platforms can be used to gather large datasets with heterogeneous properties stored using unstructured format and complex relationships between values. Deep learning approaches can be used to extract knowledge and analysis large and complex datasets.
Detection and localization of objects within images is considered an essential task in various computer vision algorithms. Various studies addressed the detection and tracking of facial landmarks including the iris and pupils which has various applications including eye gaze estimation for human machine interfaces. Composite of techniques are utilized in sequential phases while leveraging the deep learning based facial landmark detection to extract eye information within an image/video frame. Existence of background noise and dark patches within the image frame (such as eye-browse) have been identified as a major cause degrading the performance of computer vision-based iris and pupil detection. However, this issue can be resolved by utilising modern deep learning algorithms for reliable face and eye frame extraction from an ordinary quality images/video. The presentation will elaborate on the use of deep learning for image analysis as well as on new applications, trends and chances.
Partners
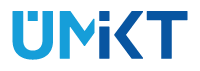
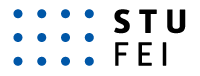
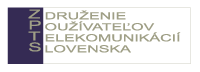